Resource Center
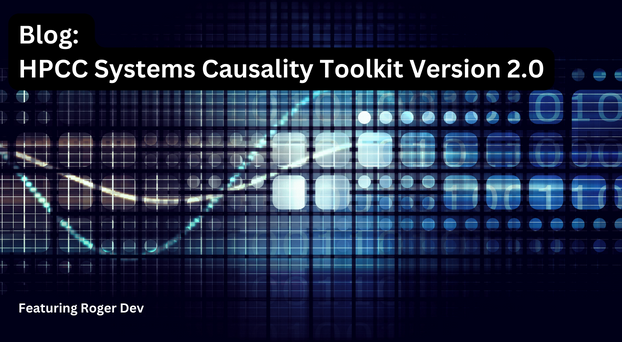
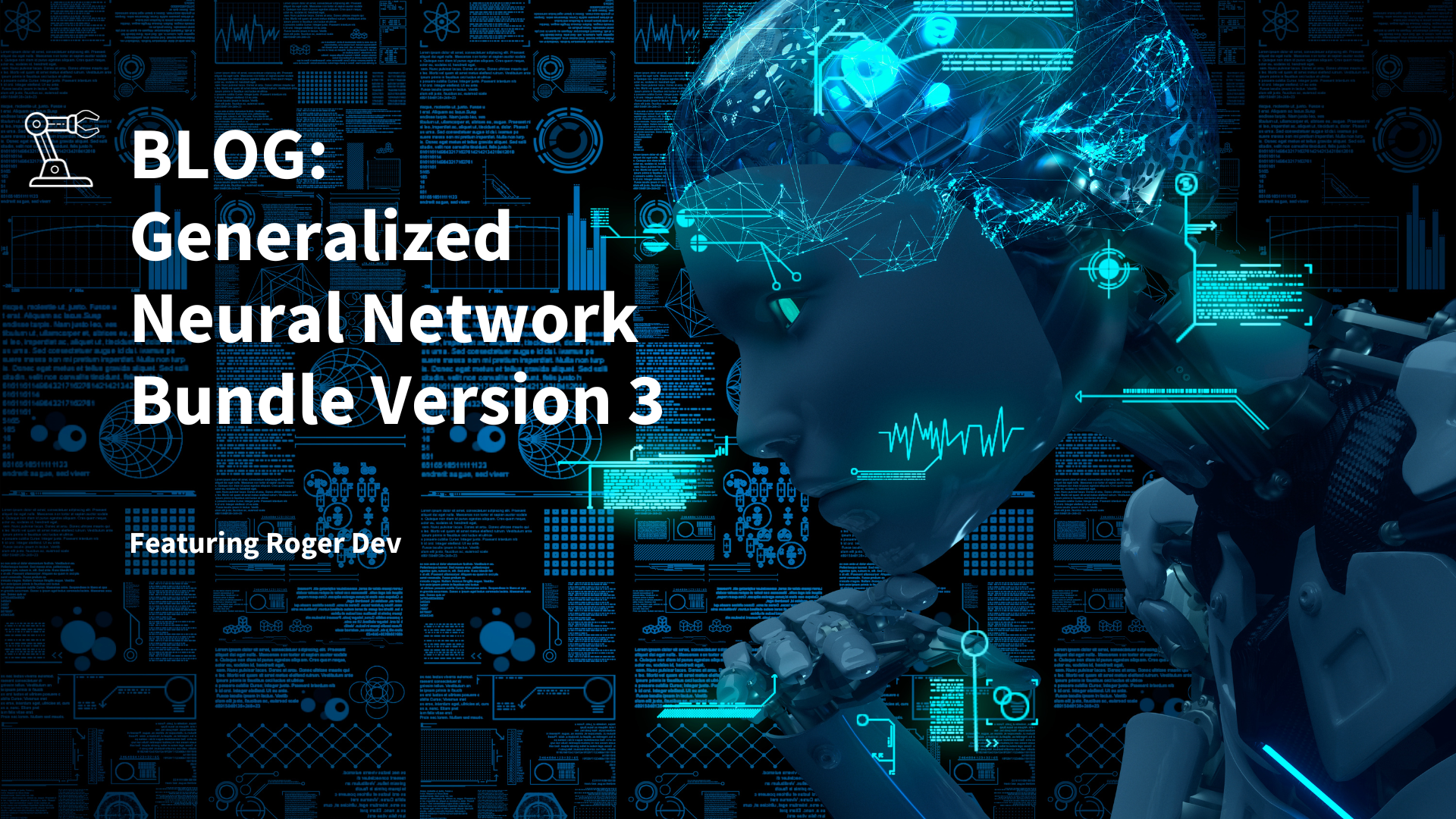
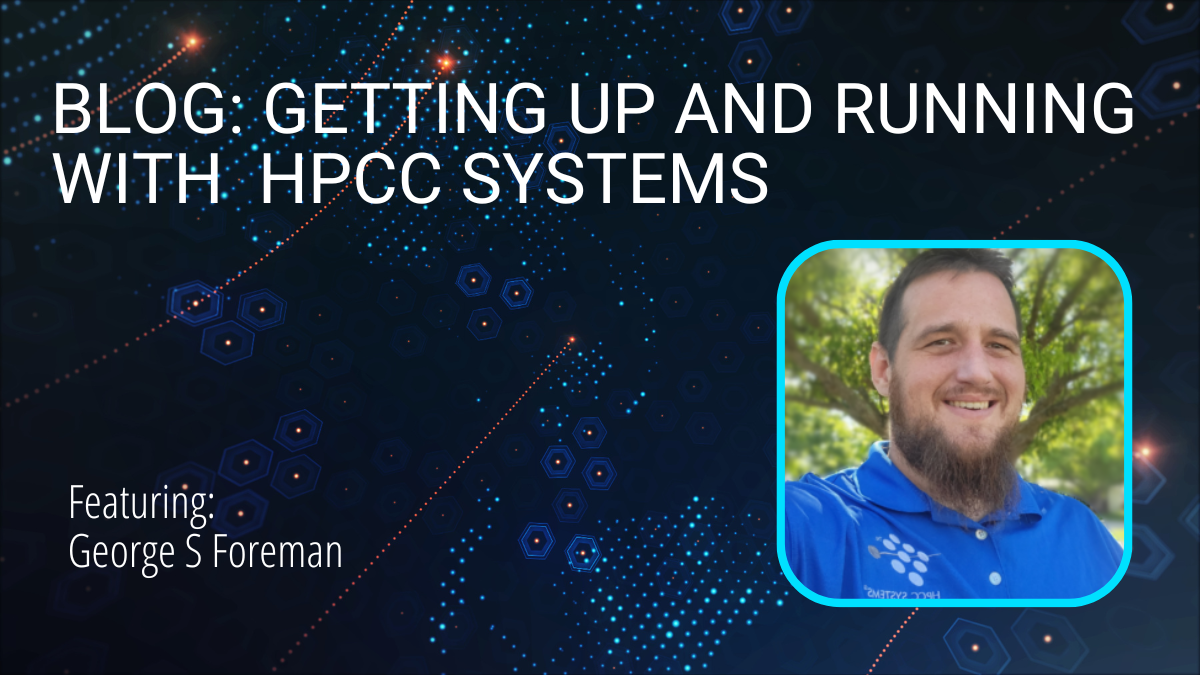
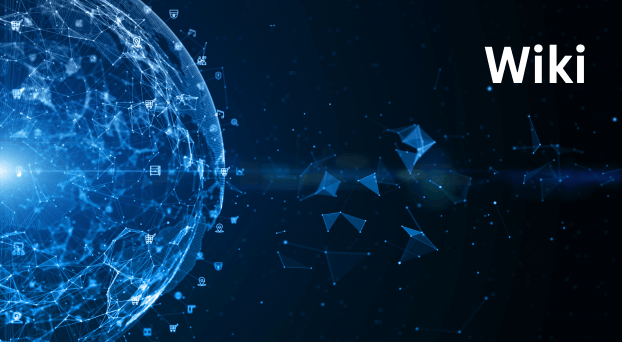
HPCC Systems Interactive Expo: Meet the Machine Learning Team
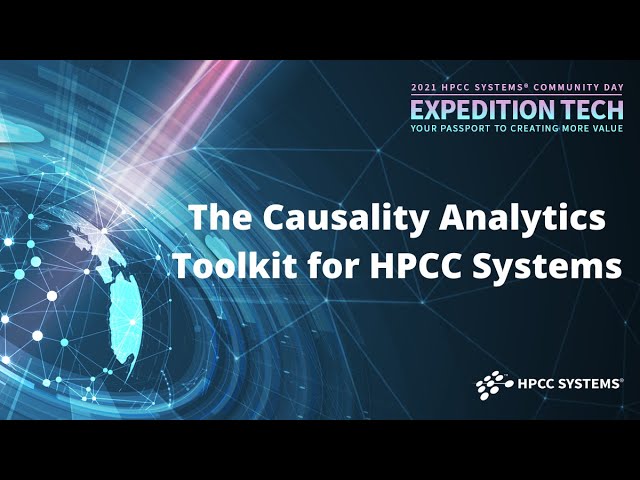
2021 HPCC Systems Community Virtual Summit: The Causality Analytics Toolkit for HPCC Systems
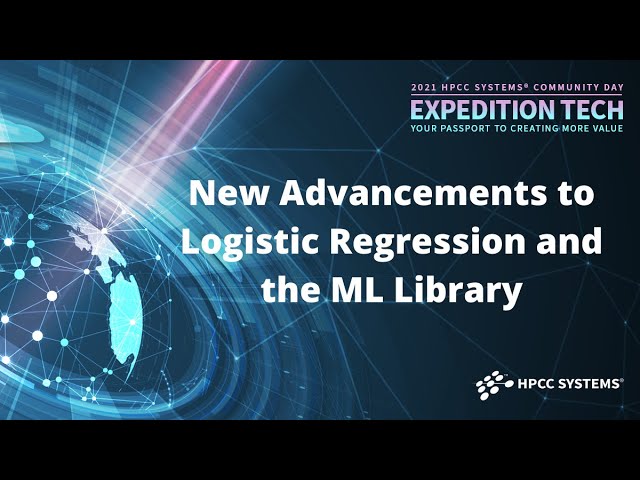
2021 HPCC Systems Community Virtual Summit: New Advancements to Logistic Regression & the ML Library
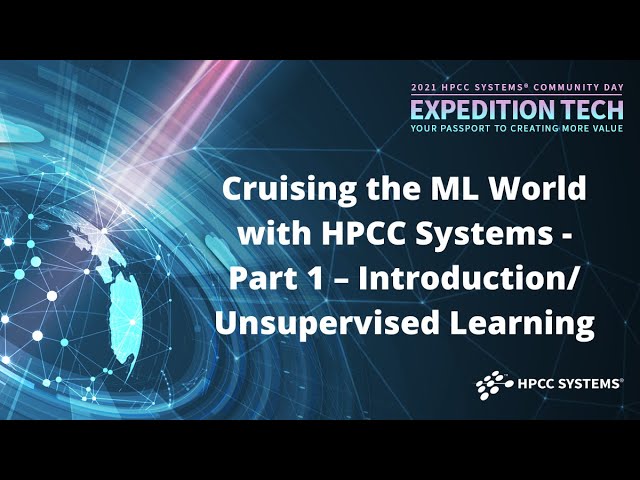
Cruising the ML World with HPCC Systems Part 1 – Introduction/Unsupervised Learning
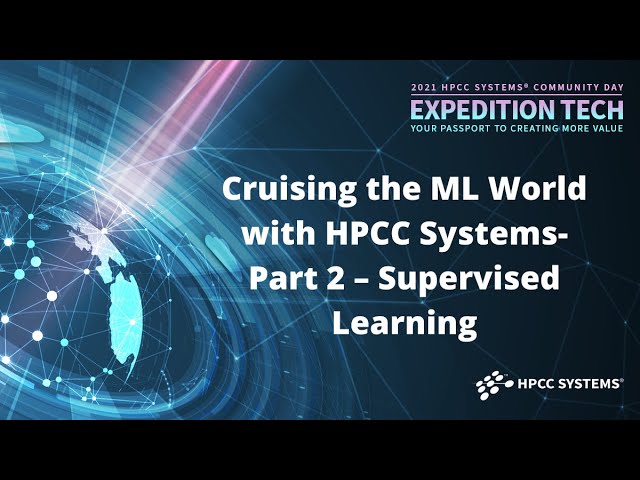